Fugatto from NVIDIA represents one of these groundbreaking AI applications; its rapid advancement is revolutionizing audio synthesis and transformation technology. Recently, their team released Fugatto as an audio transformation model capable of responding to freeform text commands to produce highly customized audio outputs. In this article we provide an in-depth explanation of its core concepts, major challenges and immense potential of Fugatto technology.
Fugatto Concept Overview
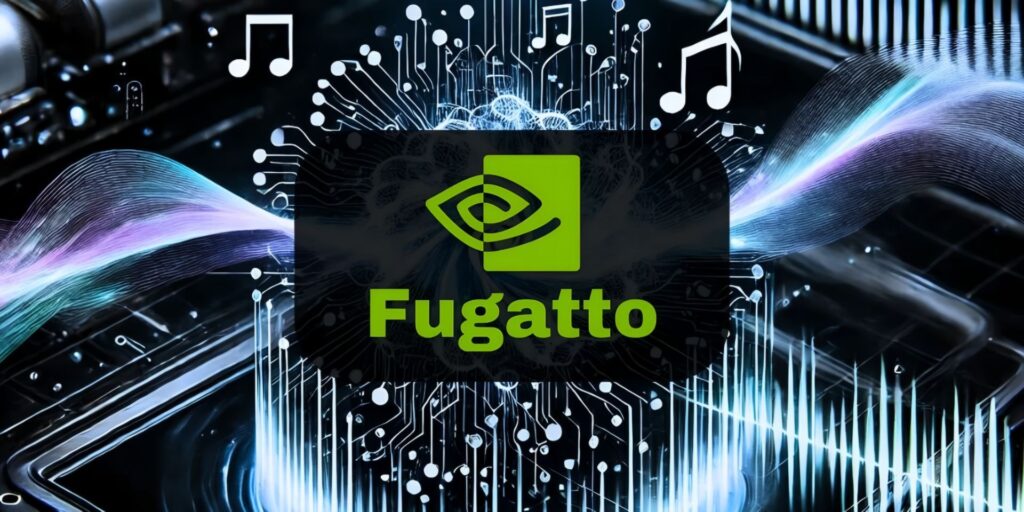
Fugatto excels at versatility and flexibility. Traditional audio processing models tend to focus on specific tasks; Fugatto stands out as being a general-purpose tool for audio generation and transformation, capable of both text-driven generation as well as performing various transformations such as merging, interpolating or negating specific commands.
Fugatto harnesses large datasets and sophisticated machine learning techniques to meet its objectives. While traditional models typically require specific tuning or configuration for every task, Fugatto’s design enables it to adapt easily to diverse audio generation and transformation requirements – an invaluable asset for audio engineers, creatives, game developers and casual users looking to explore sound.
Traditional models tend to excel at one specific task while becoming useless when confronted with data or task variations, however Fugatto stands out by operating across various tasks without impacting performance. This unique capability stems from its extensive understanding of audio/linguistic relationships; paying special attention to how various instructions alter sound synthesis.
Overcoming Command Generation Challenges
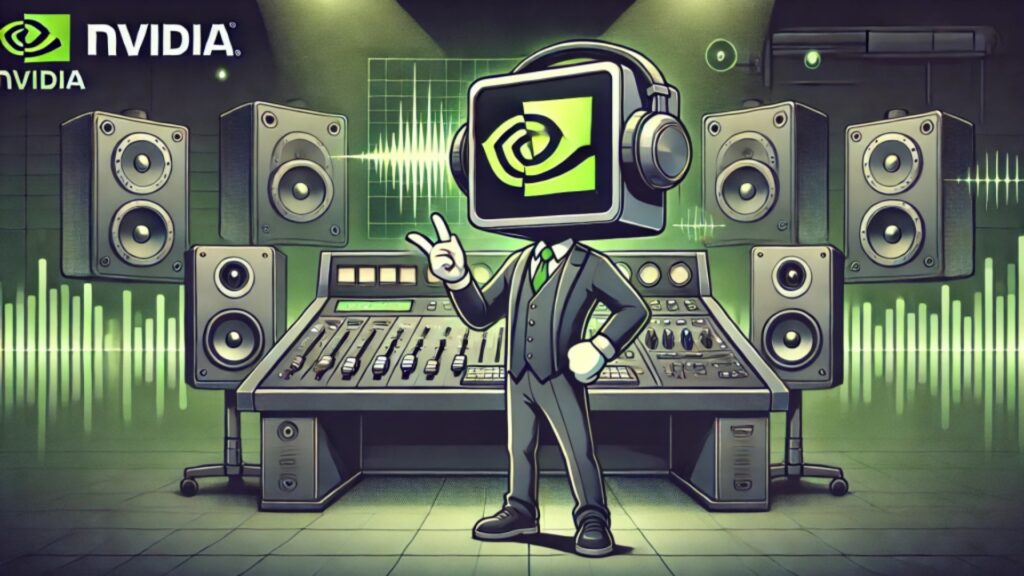
Fugatto presents numerous challenges when creating audio data; one such obstacle lies in audio data’s inherently lacking command information that was used to generate it compared with textual data where large language models (LLMs) can deduce instructions directly from written words. To address this obstacle, researchers created a specialized dataset generation method; with it comes various audio tasks which create meaningful correlations between language and audio data sets.
The process for data generation entails several essential steps:
Utilizing LLMs for Instruction Generation
By employing large language models to generate and augment instructions and captions, Fugatto learns how to respond appropriately to different user inputs. This makes its dataset richer with more natural-sounding language commands that enhance Fugatto.
Generating Absolute and Relative Instructions
Researchers created instructions that can either be absolute (e.g. “synthesise a happy voice”) or relative (e.g. “increase happiness of this voice”). This dual approach enables Fugatto to effectively handle dynamic tasks while making adjustments on-demand to audio properties.
Leveraging Audio Understanding Models
y employing audio understanding models to generate descriptions and synthetic captions for audio clips, annotating data becomes much richer, which improves its generalization capabilities and performance even in situations with minimal annotated material. This increases generalization capabilities while simultaneously increasing performance under conditions where annotated information is scarce.
Transforming Existing Datasets
Our team explored methods to modify and enhance existing datasets in order to reveal new relationships between text, audio and their transformations – providing opportunities for creating entirely new tasks without needing more raw data; optimizing resource use.
Fugatto relies heavily on data that is sufficiently rich and varied in order to create an expansive training ground for its neural net model, Fugatto. Fugatto utilizes this robust dataset as the cornerstone for generalized audio outputs across numerous environments based on diverse instructions.
Reaching Breakthroughs in Compositional Abilities
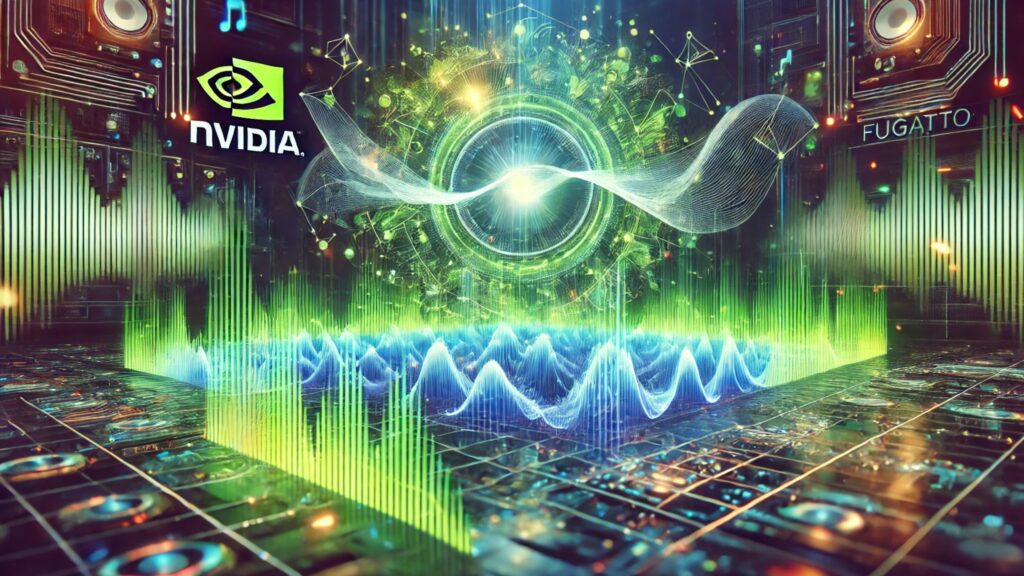
Fugatto faces another significant difficulty when handling combinational commands, meaning more complex instructions like merging multiple commands together or interpolating between two of them. To address this challenge, researchers developed an inference technique known as ComposableART that helps manage these complex instructions more easily.
ComposableART (Composable Audio Representation Transformation) is an innovative method that extends classifier-free guidance during inference, providing flexible composition of instructions. This allows the model to produce highly customizable audio outputs. Users may instruct ComposableART to combine characteristics from multiple samples into one output or negate certain features to produce their desired outcome.
ComposableART plays an essential part in Fugatto’s adaptability. By permitting instructions to be composed and decomposed with ease, Fugatto can handle scenarios where users need to refine or adapt their commands iteratively – something especially helpful in creative fields such as music production or sound design, where expressive flexibility is an absolute requirement.
ComposableART’s advanced sound creation tools enable artists and engineers to explore sounds previously out of reach; its seamless merging, adjustment and reformulation capabilities create an expanded sonic palette enriching creative processes and expanding imaginations alike.
Enhancing Dataset Diversity
Fugatto’s robust performance across various tasks was ensured through an array of data and command generation strategies implemented by its researchers:
Using large language models to generate and augment instructions and captions
Doing this enables the model to learn natural-sounding commands closer to free form speech, increasing its understanding and following user inputs more closely.
Developing both absolute and relative instructions
Instructions such as, “synthesize a happy voice,” or, “increase its happiness” allow models to adapt dynamic tasks easily by making instantaneous adjustments of audio properties on-the-fly.
Applying audio understanding models to generate descriptions and synthetic captions of audio clips
By enriching the dataset with meaningful annotations – especially where annotated data is scarce -, an audio understanding model’s generalization and performance improves significantly.
Transformation of existing datasets to identify relationships
This approach maximizes resource use efficiency by permitting creation of tasks without additional raw data requirements.
By combining various approaches, researchers ensured Fugatto had access to an expansive and varied dataset, which enabled him to learn across audio domains and contexts – providing the foundation for unsupervised multitask learning at scale as well as uncovering emerging abilities like synthesizing entirely new sounds. This unique combination allowed Fugatto access to an unparalleled dataset enabling unsupervised multitask learning at scale as well as unlocking emergent abilities like synthesizing entirely novel sounds.
Fugatto’s Real-World Performance
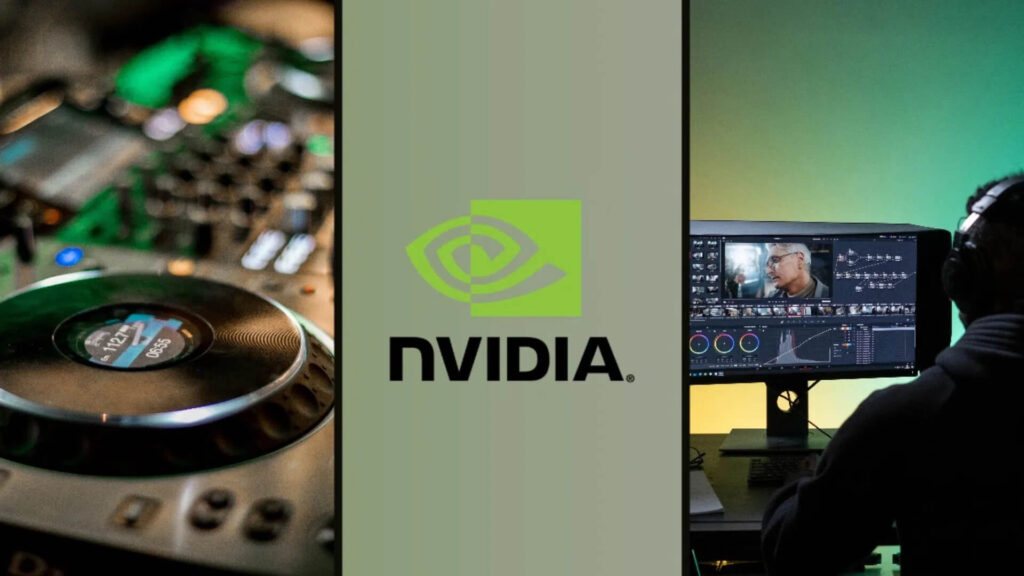
Fugatto has demonstrated competitive performance compared to specialized models optimized for specific tasks, in various tests and tasks. From producing audio from scratch based on text descriptions or transforming existing audio in highly specific ways to creating brand new tracks from existing tracks; Fugatto takes these challenges with great agility.
Fugatto stands out amongst other models for its extraordinary capacity to generate unique sounds, thanks to ComposableART. Fugatto can produce audio that has never before been heard; for instance, using this model one may instruct it to generate a saxophone tone which mimics dog barks as evidence of its extraordinary creative capacity.
Fugatto’s versatility extends across various application fields. Music production uses it to help artists and producers craft unique soundscapes and effects; gaming uses it to generate immersive and dynamic audio environments; virtual reality utilizes it to provide realistic yet context-sensitive soundscapes which enhance user experiences – the possibilities are virtually limitless!
Fugatto stands out in both educational and research settings. For instance, its use can help study how certain sounds impact emotions or behavior in humans – providing invaluable insights in fields like psychology and cognitive science. Furthermore, its capability of producing high-quality audio through diverse and complex instructions makes Fugatto an excellent language learning tool, offering students an engaging way to improve listening comprehension abilities through immersive interaction and engagement.
Conclusion
Fugatto and ComposableART technologies from NVIDIA represent groundbreaking innovation in audio synthesis and transformation technology, opening new avenues of application within creative fields and beyond. As this technology progresses further, its potential could spread even wider.
As Fugatto becomes more widely adopted and its capabilities refined, we can anticipate even more remarkable advances in audio technology. From creating entirely new genres of music to building immersive virtual reality soundscapes – Fugatto promises to revolutionize how we experience and think about sound – making its presence felt now more than ever – the future is truly here – it sounds incredible.
Fugatto represents an impressive achievement in audio technology. By seamlessly blending cutting-edge machine learning techniques with intuitive understanding of language and audio synthesis/transformation tasks, NVIDIA has produced a tool which not only meets but exceeds contemporary demands in terms of synthesis/transformation tasks; and as we look ahead to its continued refinement it’s apparent this groundbreaking model will play an essential role in shaping its future development and improvement.
The content of this article is based on an interpretation of the paper “Fugatto 1-Foundational Generative Audio Transformer Opus 1”. If you wish to gain a deeper understanding, you can directly read the paper.